Problem
Discovering vulnerabilities in an IoT ecosystem is like finding a needle in a haystack, even when we are dealing with known vulnerabilities. For many IoT products, security is an afterthought. Between copy-paste coding practices and outsourcing of functionality to untrusted third-party libraries, the development process of IoT devices is a fertile environment for bug generation and persistence. As several integration vendors may rely upon the same subcontractors, tools, or SDKs provided by third-party vendors, bugs generated during the development process can be spread across hundreds or even thousands of IoT devices with similar firmware. Without detailed knowledge of the internal relationships between these vendors, it is impossible to track the same vulnerability across the IoT ecosystem.
Finding vulnerabilities in devices from the Internet of Things (IoT) ecosystem is more crucial than ever. Unlike in PCs or mobile phones, a security breach in one IoT device could cause unprecedented damage to our daily life, involving massive breakdowns of public systems [1] or quality of life issues. Gartner, Inc. forecasts that 6.4 billion connected things will be in use worldwide in 2016, up 30 percent from 2015, and will reach 20.8 billion by 2020. The vast diffusion of devices will increase the potential for the introduction of vulnerabilities to the IoT ecosystem. The study by Cui et al. [2] showed that 80.4% of vendor-issued firmware is released with multiple known vulnerabilities, and many recently released firmware updates contain vulnerabilities in third party libraries that have been known for over eight years. As a result, the need for third-party evaluators (e.g., consumer product evaluators, penetration testers) to quickly and accurately identify vulnerabilities in IoT ecosystem devices on behalf of customers and the need to support periodic security evaluations on existing devices is increasing dramatically [3].
Solution
In this article, we show how to use Dr. Binary to quickly identify known vulnerabilities in IoT devices. Dr. Binary is a code analytic engine that utilizes the latest code search technology. We treat vulnerability as one or more functions. Then the problem of known vulnerability search is converted into the problem of searching semantically-equivalent functions in binary code. We first use Dr. Binary to build the vulnerability database, and then we use Dr. Binary to scan the target executable code against the vulnerability database
Vulnerability Database Preparation
We collected 92 CVEs from Bluetooth drivers, camera drivers, etc. We identified the vulnerable function for every CVE and upload it to Dr. Binary to generate the vulnerability database. Table 1 is a list of the collected CVEs.
├ CVE-2017-0781 ├ CVE-2017-0782 ├ CVE-2017-0783 ├ CVE-2017-0785
├ CVE-2016-0830 ├ CVE-2016-2439 ├ CVE-2016-3744 ├ CVE-2017-0781
├ CVE-2017-0782 ├ CVE-2017-0783 ├ CVE-2017-0785 ├ CVE-2016-0826
├ CVE-2016-2449 ├ CVE-2016-0830 ├ CVE-2016-2439 ├ CVE-2016-3744
├ CVE-2017-0782 ├ CVE-2017-0783 ├ CVE-2017-0785 ├ CVE-2016-0830
├ CVE-2016-0838 ├ CVE-2016-0841 ├ CVE-2016-2476 ├ CVE-2016-2416
├ CVE-2016-2495 ├ CVE-2016-3754 ├ CVE-2016-3861 ├ CVE-2016-3915
├ CVE-2016-3921 ├ CVE-2016-2430 ├ CVE-2016-2476 ├ CVE-2017-0781
├ CVE-2017-0783 ├ CVE-2017-0785 ├ CVE-2016-2476 ├ CVE-2016-2495
├ CVE-2016-2439 ├ CVE-2016-3744 ├ CVE-2017-0781 ├ CVE-2017-0782
├ CVE-2017-0783 ├ CVE-2017-0785 ├ CVE-2017-0495 ├ CVE-2017-0781
├ CVE-2017-0782 ├ CVE-2017-0783 ├ CVE-2017-0785 ├ CVE-2016-0830
├ CVE-2016-2439 ├ CVE-2016-3744 ├ CVE-2017-0781 ├ CVE-2017-0782
├ CVE-2016-3744 ├ CVE-2017-0782 ├ CVE-2017-0783 ├ CVE-2016-0830
├ CVE-2016-0830 ├ CVE-2016-2439 ├ CVE-2016-3744 ├ CVE-2017-0781
├ CVE-2017-0782 ├ CVE-2017-0783 ├ CVE-2017-0785 ├ CVE-2016-0830
├ CVE-2017-0781 ├ CVE-2017-0782 ├ CVE-2017-0783 ├ CVE-2017-0785
├ CVE-2016-2476 ├ CVE-2016-2430 ├ CVE-2016-3915 ├ CVE-2016-2449
├ CVE-2016-0838 ├ CVE-2016-2495 ├ CVE-2016-3921 ├ CVE-2016-3861
├ CVE-2016-2416 ├ CVE-2016-2476 ├ CVE-2016-0841 ├ CVE-2017-0386
├ CVE-2016-3754 ├ CVE-2016-2430 ├ CVE-2016-3915 ├ CVE-2016-2449
├ CVE-2016-2416 ├ CVE-2016-2476 ├ CVE-2016-0841 ├ CVE-2017-0386
├ CVE-2016-0838 ├ CVE-2016-2495 ├ CVE-2016-3921 ├ CVE-2016-3754
Table 1 CVE list
Executable code collection.
We collected 56 executable files [4] from IoT firmware images. They are bluetooth drivers, camera drivers, etc. Those libraries are popular in IoT devices.
Result
We used Dr. Binary to scan those 56 executable files [4] and found 87 vulnerabilities in 56 executables files. One thing to mention is that the discovered vulnerable functions in executable code are not always the same as the one in the vulnerability database. This may be caused by different compilation options, compiler versions, etc. But Dr. Binary still can handle it. For instance, Dr. Binary identifies CVE-2016-2439 in 14-files-bluetooth.*. The vulnerable function stored in vulnerability database is listed in Figure 1. The function Dr. Binary found in 14-files-bluetooth.* is listed in Figure 2. They are not exactly the same, and the registers at the beginning are different. The patched function is shown in Figure 3. It adds additional check for pin_code. The function found in 14-files-bluetooth.* doesn't do this. So it is indeed the vulnerable function CVE-2016-2349. This case demonstrates that Dr. Binary can tolerate compilation variances.
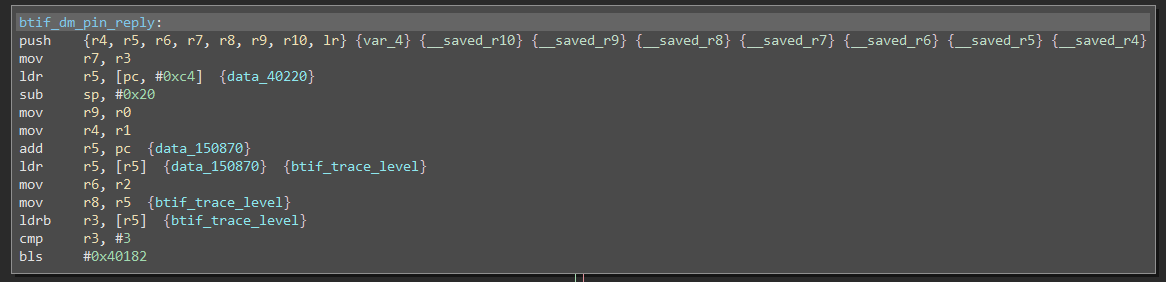
Figure 1. CVE-2016-2349 in vulnerability database
Figure 2. Vulnerable Function found in 14-files-bluetooth.*
Figure 3. Patched Function
Summary
This article demonstrates the usage of Dr.Binary in finding vulnerabilities in IoT devices. It collected 92 CVEs and 56 executable files. Finally, it identifies 87 vulnerabilities in 56 executable files. Although the CVEs are old, but it still exists in IoT devices we investigated. Interested readers can click
here to try Dr. Binary.
Reference
[1] Internet of things: When cyberattacks have physical effects. https://www.federaltimes.com/
opinions/2016/04/08/internet-of-things-when-cyberattacks-have-physical-effects/.
[2] A. Cui, M. Costello, and S. J. Stolfo. When firmware modifications attack: A case study of embedded exploitation.
In NDSS, 2013.
[3] Cybersecurity and the internet of things. http://www.ey.com/Publication/
vwLUAssets/EY-cybersecurity-and-the-internet-of-things/$FILE/
EY-cybersecurity-and-the-internet-of-things.pdf
[4] https://drive.google.com/drive/folders/1stTOrqtZVbyxTLW8qz4pTHyFVWnLg6Dh?usp=sharing
Well, the selection is relative to particular person taste, so why not simply enjoy each of the features made available at Betiton™? Here are the professionals and cons of the live and digital format of roulette. The proof is within the pudding, as they say, 카지노사이트 and here at Betiton™, might have} the best online roulette UK gamers might wish to experience.
ReplyDelete